In the race to harness the transformative power of AI, the true battleground lies in inference—the stage where trained models are deployed to make real-time decisions. Reducing compute costs is the key to unlocking AI’s full potential.
Over the past year, generative AI, driven by transformer models, has demanded significant compute power. Yet, its adoption remains limited as enterprises explore its capabilities. High compute costs, driven by the training phase, are a barrier. Once integrated into workflows, the focus shifts to inference, altering the cost dynamics for any organization incorporating AI into their business operations.
The Insatiable Demand for Inference
Infrastructure that is purpose-built and optimized for AI is no longer a luxury—it’s a necessity. Currently, the costs associated with compute, especially for inference—the stage where an AI model is put to use—are prohibitive. To catapult AI into the mainstream, better compute economics and efficiencies are not just desired; they are desperately needed.
As illustrated in the chart below, while the initial training costs for AI/ML models are significant, they are relatively short-lived. The real challenge lies in the production phase, where inference costs continue to rise dramatically throughout the year, eventually dwarfing the training costs.
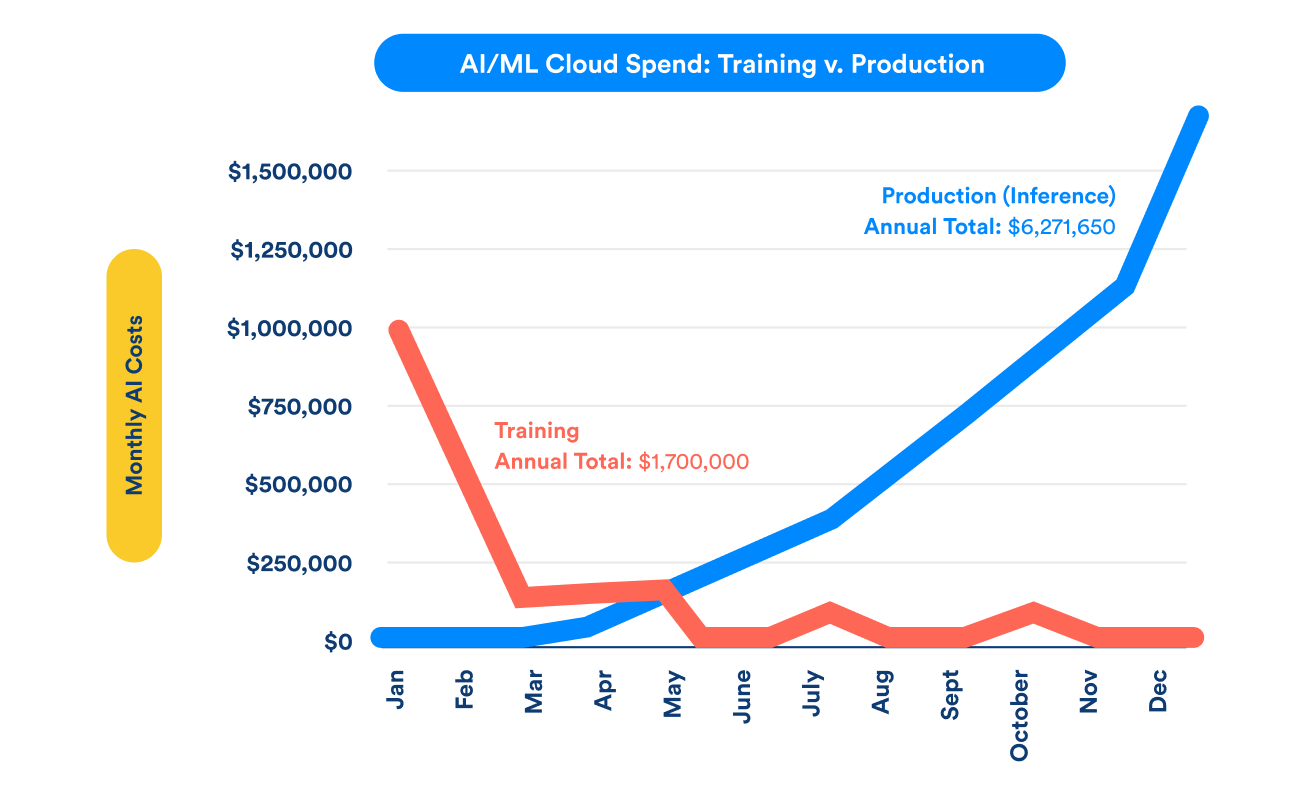
Source: Jaya Plmanabhan
As the use of large language models becomes more widespread, we are witnessing an uptick in AI-dedicated servers. Over time, inference costs—recurrent costs of running these models after they’ve been trained—begin to dwarf the initial training costs. As generative AI gains traction across industries, inference costs are projected to surpass training costs.
Today’s Status Quo
Nvidia General Processing Units (GPUs) are extremely high-powered chips currently in demand for the high-intensity training requirements of new AI models. Public market investors have certainly taken notice, with Nvidia’s stock rising more than 700% since the public debut of ChatGPT in November 2022. However, when used for inference compute, these chips are overkill.
Normally, extra compute is not a bad thing. However, the current gating factor around data center computing is the power and energy efficiency of the chips themselves. To fit more computing power into a data center, we are now left with two options: change the existing cooling infrastructure or build a more energy-efficient chip.
Enter: d-Matrix
Recognizing this, d-Matrix is not casting a wide net but is instead meticulously constructing specialized solutions for the AI inference market. Their strategic position is to complement, rather than compete with, incumbents in the training compute sector. While software solutions exist to alleviate these issues somewhat, their effectiveness is often limited. It’s at the silicon level where we see the most significant opportunity for impact—each incremental improvement here can lead to outsized gains in efficiency and cost-effectiveness.
Everything begins with silicon, the beating heart of technology. Current CPUs and GPUs were not architected with the intricate structure of AI models and processes in mind. AI-specific chips have emerged to rectify these inefficiencies, offering faster parallel processing, higher bandwidth memory, and improved power efficiency.
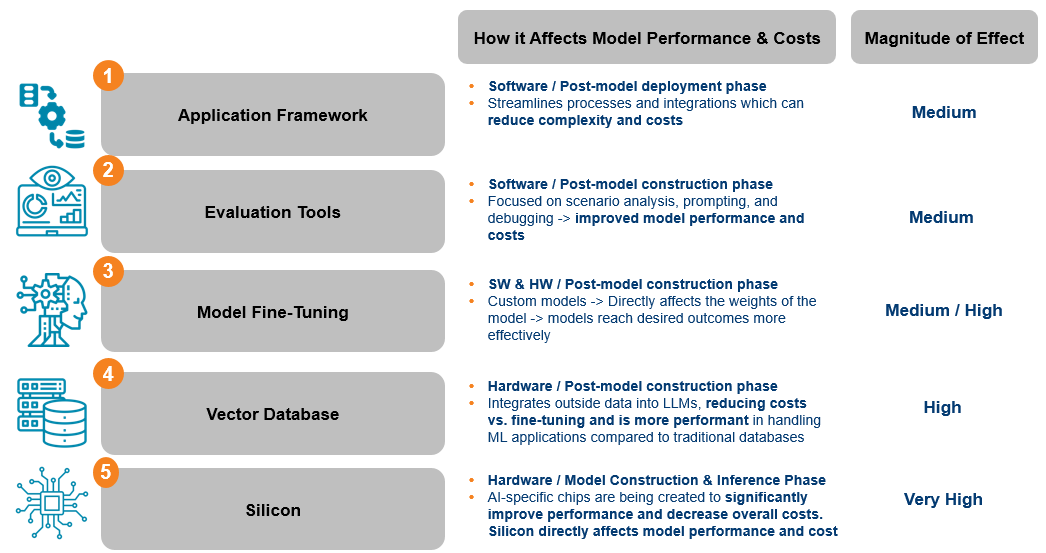
Source: Mirae Asset Research
d-Matrix has distinguished itself as a silicon company targeting AI & Transformer models and inference. Their innovative architecture and platform have been engineered from scratch, specifically for Generative AI and Transformer models. They offer a best-in-class solution that is not only power-efficient but also more cost-effective for inference computation than industry giants such as NVIDIA.
The technological prowess of d-Matrix is encapsulated in two key differentiators: their in-memory compute (IMC) architecture, which minimizes data movement, reducing latency and power consumption, and their focus on chiplet architecture, which provides unparalleled optimization and flexibility for customers.
In the race for the most power-efficient inference chip solution, d-Matrix is leading the pack.
A Future with AI Ubiquity
With AI models becoming integral to industries like healthcare, finance, and autonomous driving, the demand for efficient inference solutions is skyrocketing. d-Matrix is poised to lead this transformation, making AI accessible and affordable for enterprises of all sizes.
Our investment in d-Matrix is a strategic move to capitalize on the AI revolution. By addressing the critical challenge of inference compute costs, d-Matrix is set to become a cornerstone in the AI infrastructure landscape. For us, the decision was clear: investing in d-Matrix is investing in the future—a future where AI is ubiquitous, efficient, and economically viable for all.
To learn more, please reach out to our team at US.Venture@miraeasset.com